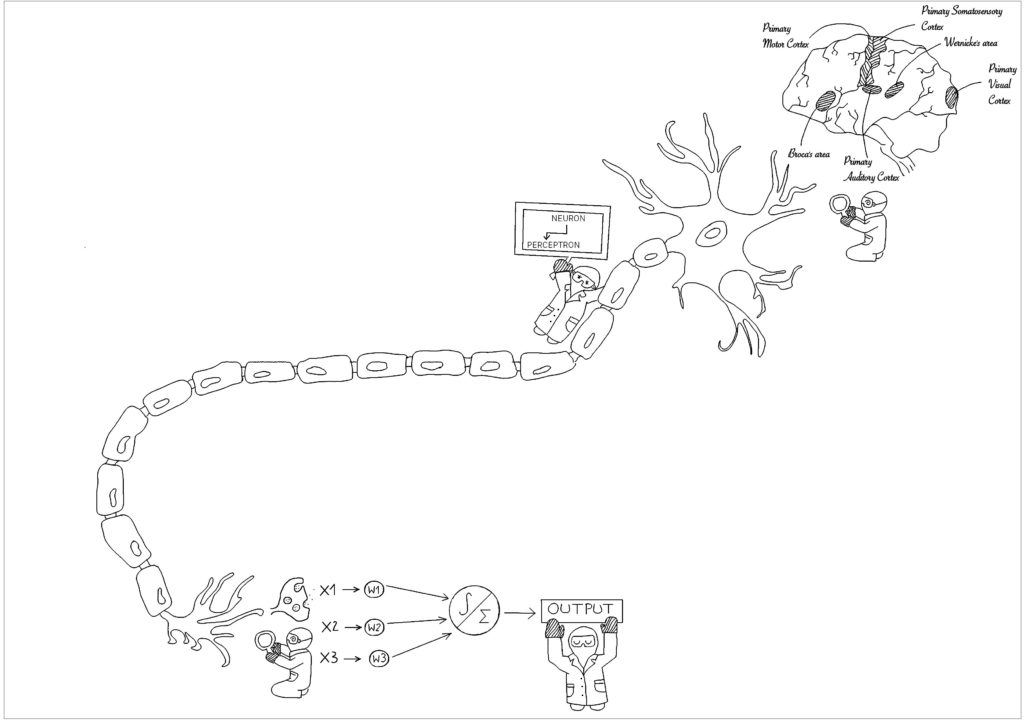
Every day our brain is exposed to a massive input of information to be processed. Not all information is as important to perform a certain task – just as unlocking your phone does not necessarily require you to use complicated algebra. The ability to form certain connections when necessary is an ability that our brain learns to master with time. Therefore, it is categorized into functional regions. The connections between those regions can be studied on a functional level that indicates neuronal activity. One technique to do so is functional Magnetic Resonance Imaging (fMRI) developed in the 1980s and ’90s (Power et al, 2010). It is used to monitor brain activity using the blood oxygen level-dependent (BOLD) single changes with which neural activation can be captured. By following changes in the brain activity in any given situation, specific regions could be identified that are associated with critical functions such as listening and speaking, seeing and remembering, or sensing and responding (Raichle, 2010).
The development over time and the spatial location associated with the functional manifestation of certain brain regions can convey different information throughout the human lifespan. Functional regions such as the amygdala associated with fear and anger, the hippocampus associated with memory processing, and Wernicke’s and Broca’s associated with speech are just some examples supporting the understanding of how our brain is organized. Following the question of how specific brain regions are connected, fMRI could be used during a resting state to compare functional connectivity from infant to child- and adulthood (so-called rs-fcMRI). Studies suggested that at younger ages the rs-fcMRI signal correlation between nearby brain regions is stronger and that with adulthood those signal correlations are again found between brain regions further away. Although the data do not allow conclusions, it indicates changes in functional connectivity during a life span.
The increase in brain regions over time is primarily found within structures involved in task control and the default network (Power et al, 2010). As our behavior and cognitive ability change while we grow up, so does our brain architecture adapt to the demands of living. This can be influenced by many factors such as the environment, social circumstances, or epigenetics. Concepts such as synaptic pruning or synaptic plasticity can contribute to drawing the neural network as the brain learns to adapt with time. Keeping that in mind, to understand a network we would need to study the relationships between the entities involved. This means to look at the level of neurons as entities that are connected to build up a complex network determining our brain function. Central to the connection of neurons are synapses which are coupled to the release of neurotransmitters from the pre-synaptic neuron to the postsynaptic neuron if a certain threshold of the action potential is reached.
Fascinating is the transfer of biological knowledge on a computational level, as the overlapping principles started to be expressed in the mathematical modeling of neuronal activity in 1943 (Géron, 2019, O’Riley). Starting from propositional logic, neurophysiologists Warren McCulloch and mathematician Walter Pitts joined forces to describe the idea of calculating the activity between biological neurons. The work was picked up by researchers but lost focus as low data volume and decreasing financial support prevented progress. Nowadays, the quantity of data increases constantly and the computing capacity improved to a point where the training of huge artificial neural networks produces promising results within a reasonable timeframe. The more we understand the brain and its connectivity, the better we can apply the knowledge to the field of artificial intelligence. One of the first architectures of a neural network was described in 1957 by Frank Rosenblatt as a perceptron. Perceptrons serve as a computational equivalent to a biological neuron which is still the foundation for present complex multilayer neural networks as a representation of multiple layers found in the biological neural network of the brain (Géron, 2019, O’Riley). Findings using rs-fcMRI showed the changes in the correlations between the Default Mode Network of certain brain regions between children and adults which mainly increase especially from the front to the back of the brain (Power et al, 2010). The role and maturity of the system at any given age are still under investigation, and the findings could provide a better idea of how the network evolves with time. Questions of how the information of functional connectivity from the human brain can be transferred and useful to the system of artificial neural networks are yet to be explored. Convolutional neural networks are an example where knowledge about the organization of neurons within the visual cortex was used as a point of reference and now they are applied to a wide range from image recognition within the medical field to drug discovery or health risk assessment (Ravi et al, 2017). The progress is impressive and so is the responsibility that comes with it.
References
1. Power, J. D., et al. (2010). “The development of human functional brain networks.” Neuron 67(5): 735-748.
2. Raichle, M. E. (2010). “Two views of brain function.” Trends Cogn Sci 14(4): 180-190.
3. Ravi, D., et al. (2017). “Deep Learning for Health Informatics.” IEEE J Biomed Health Inform 21(1): 4-21.
4. Aurélien, Géron (2017). “Praxiseinstieg Machine Learning mit Scikit-Learn und TensorFlow” O’Reilly, ISBN Print: 978-3-96009-061-8